Feature Spotlight: Sampling
Tuesday, December 21, 2021 by Anthony DeJohn
The December 2021 Nebula update included several great features that will help case teams derive actionable intelligence
from their data. One of those features - Sampling - is an extremely powerful tool that allows even just one person to assess, and potentially act on, many thousands of records in very little time. But first, it's worth discussing some of the fundamentals
of sampling and why it's an invaluable technique for successful eDiscovery.
What is (random) sampling?
There are many different types of sampling that statisticians and data scientists use to analyze, categorize, and even predict data outcomes. For the current Nebula implementation, we are talking exclusively about random sampling - "simple" random sampling,
to be exact. This is a common technique used across most industries for various purposes. (Simple) random sampling is exactly what it sounds like - a completely random selection of items from some larger population (think: drawing names out of a hat)
Generating a random sample allows an analyst to create small, manageable sets that are representative of the whole. How small samples can be, and the level of assurance that any given sample is reliable, is discussed below. Most importantly, it is the true randomness of the selection that creates fundamental representation from small fractions of data sets.
One aspect of sampling that usually comes as a surprise is the sample size required to get an accurate read on things. There is often much analysis that determines sample size appropriateness for various applications, but the gist of it is this: if it's not a matter of life, death, or safety, a few thousand items is generally more than sufficient. Sampling for an unofficial but actionable peek into some data? Even a few hundred will suffice. There are plenty of applications where even a few dozen will do just fine (I do this a lot).
Another fun fact about sampling: it doesn't really matter how large the whole population is (in most cases). The representativeness of any 100-item sample is dependable, whether the whole population is 1000, 10,000, 100,000, or 1,000,000.
A few technicalities
A quick primer on sampling lingo - there are two phrases that come up a lot: confidence levels and confidence intervals (aka error margin). What do these parameters mean? An example using dentists makes this easier to illustrate:
Confidence Level = 95%
Margin of error = 5%
These parameters yield a typical sample size of 384 (dentists). If 307 out of 384 (80%) dentists agree that flossing is necessary, then my sample tells me the following:
"If I ran this dental survey 100 times, I can be sure that for at least 95 of those surveys, I'd get results that range between 75%-85% (80% +/- 5%) dentist agreement. The other five surveys would possibly yield "outliers" - results outside of the measured 75%-85% range."
To summarize, confidence level is an indication of repeatability across samples, while margin of error is an indication of variability of expected outcome within any given sample.
One final point worth mentioning is that the response distribution can have a material impact on these calculations. An even-split 50/50 distribution is something of a “worst case scenario” in terms of requiring the largest sample sizes. As such, since most analysts know very little about the outcome before the sample is drawn, distribution is generally assumed to be 50/50 as a matter of caution. But what this actually means is that the confidence interval is something “less than or equal to" the specified confidence interval.
eDiscovery Implications
Although many eDiscovery practitioners only use sampling in connection with TAR protocols, there are no limits as to when sampling can be used. Trying to decide if a few search terms are producing junk? Sample 40 documents. Interested in what CustodianA was emailing CustodianB about? Sample 20 emails. Want a reliably definitive estimate as to how many of these 1,000,000 documents are going to be privileged? Sample 500 documents.
Sample sizing in eDiscovery is a frequent topic, and one that has no single correct answer. On one hand, samples in eDiscovery rarely impact life or death, nor do they present danger to human safety. On the other hand, there may be some cost (usually strategic or financial) associated with “being wrong,” and that cost can be far greater than the cost of labeling larger samples. Regardless of the level of effort put forth, random sampling is a way to make fast but informed decisions based on probabilistic reality rather than gut feel. Small and frequent samples can dramatically change a practitioner's understanding of case data in very little time. This alone presents a huge improvement over many traditional methods of carrying out discovery tasks.
Note: for potentially tricky or adverse production/disclosure related issues, it’s recommended to engage an experienced eDiscovery Technologist to help ensure defensibility and compliance.
Nebula Sampling
As with all Nebula features, our goal with Sampling was to make it intuitive, available, and fast. Additionally, we wanted a sampling feature that was both personal and 'smart' enough to persist for as long as the user desired. Where we ended up was on the document list toolbar - any document list, to be exact.
When a document list loads, users will see this icon in the toolbar:
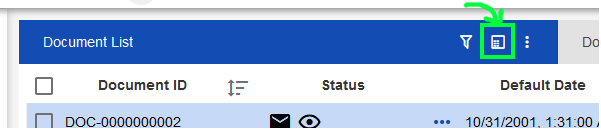
Once clicked, users are then asked to choose one of three common random sampling methods:
- Count (a specific number of documents the sample should be comprised of)
- Percentage (a specific percentage of the original document list)
- Statistical (a sample size calculation based on desired confidence level and margin of error)
Note that when a sample is generated by the user, it effectively becomes a 'virtual' search that only the user can see. That sample is intrinsically tied to the originating document list object, be it a search, batch, or list. If the user navigates away
or even logs-out, the sample will still be there. Every user-object pair can have exactly one cached sample, and those samples are retained until the user decides to delete or replace the sample.
While the sample retention feature is handy, note that samples are not directly saveable as object types, nor are they directly shareable to other users. Users that want to share or save a sample in perpetuity should bulk associate to a List, and work from that List going forward.